A Practical SEO Guide to User Intent
The whole SEO industry has been debating how best to understand what the searcher's intent is for the past few years. In this guide, we look at answering practical questions around user intent, what it is, how to analyse it and what to do with your findings.
Other Ultimate Guides
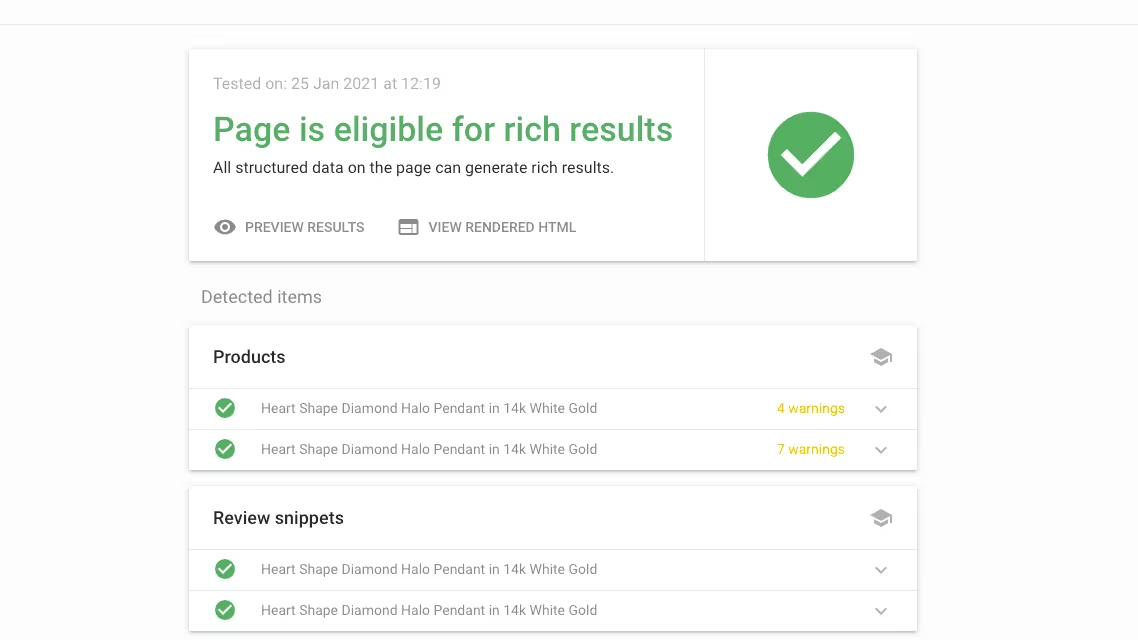
A Practical SEO Guide to eCommerce SEO
If you would like to drive more buyers to your eCommerce site, then you need to learn the most successful techniques for growing relevant traffic to your site using a combination of SEO techniques and tactics. In this actionable guide to eCommerce SEO, we cover some of the unique characteristics of optimising for online stores that differ from non-transactional sites.

A Practical SEO Guide to Backlinks and Link Building
Everyone says 'Content is King' but links have always been a critical part of Google's ranking algorithm and are likely to remain as important in the future. So, dive in and pick up some great backlink analysis and link building techniques.
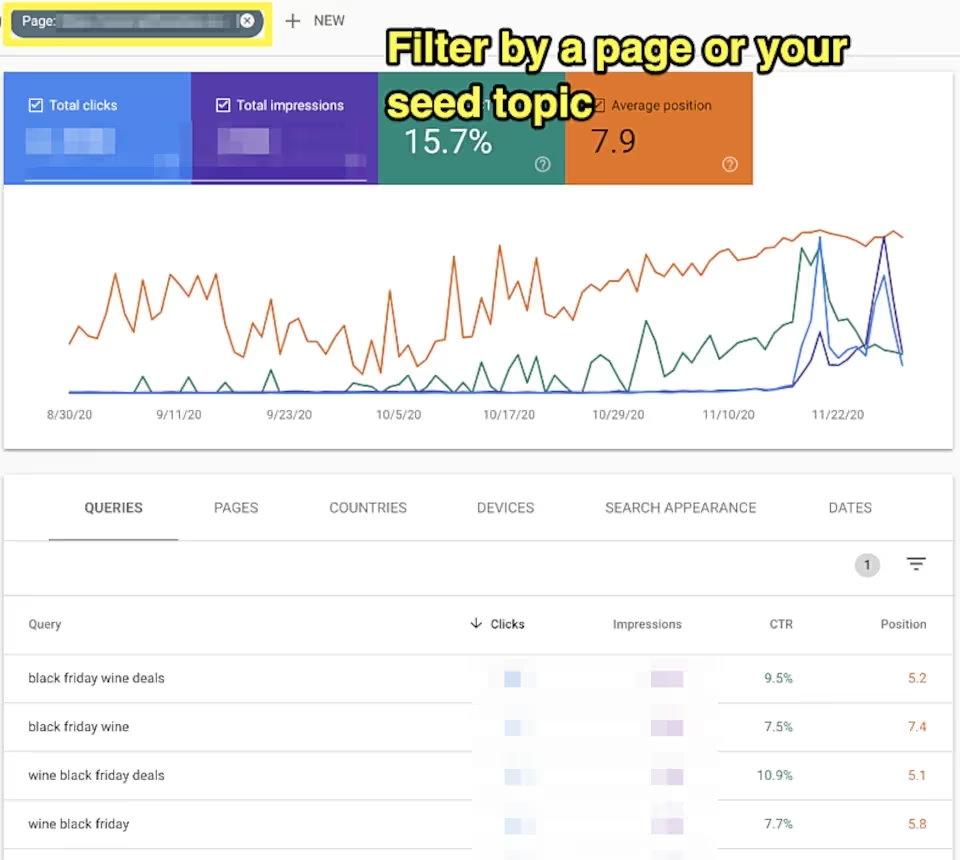
A Practical SEO Guide to Keyword Research
Keyword research is a foundational and essential part of any SEO strategy. In this extensive guide, the authors take you through the keyword and audience research process, data collection techniques, competitive gap analysis and how to interpret user intent and make decisions around page mapping to keyword groups. Use this guide to develop a systematic approach to keyword research that allows you to set your SEO efforts in the right direction.

A Practical SEO Guide to SEO Reporting
Whether you are part of an enterprise in-house SEO team or SEO agency team it is more likely than not that you will have to ‘report’ on and share your SEO progress and activities with management, colleagues or clients. This guide discusses the type of metrics to report and then different ways of producing high quality insightful SEO reports.
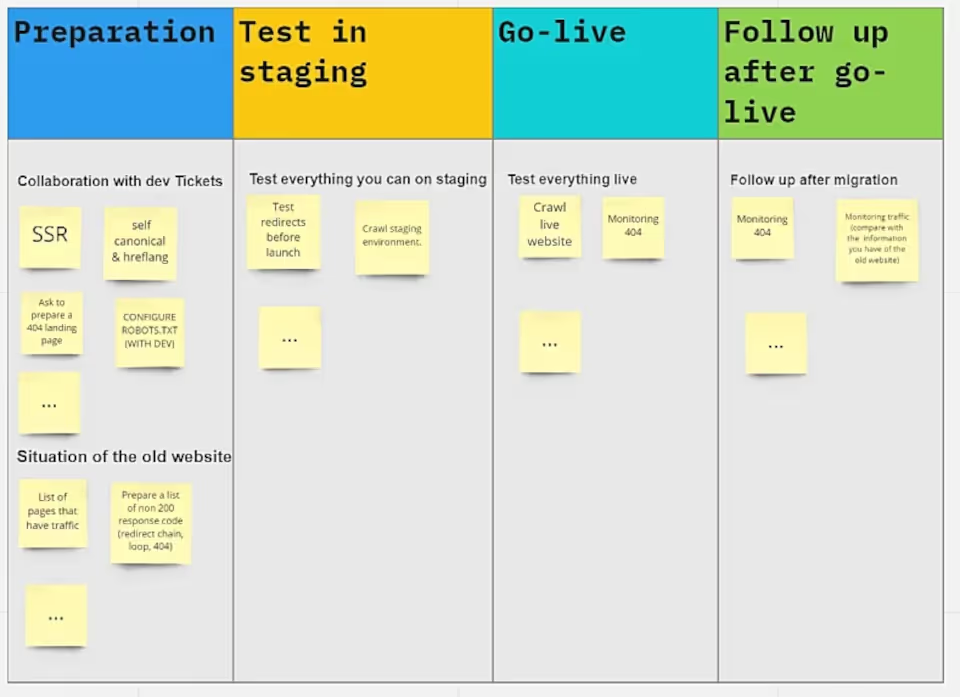
A Practical SEO to Website Migrations
Probably one of the most stressful and time-consuming tasks that we all have to face at one stage in our career is to manage a website migration project. Whether this is due to re-branding, an acquisition or merger, a change of domain name or a change of technology platform, there is an awful lot that can (and often does) go wrong. In this guide, three SEOs with extensive experience of website migrations cover the essential steps in planning and executing the site build before and post-launch.
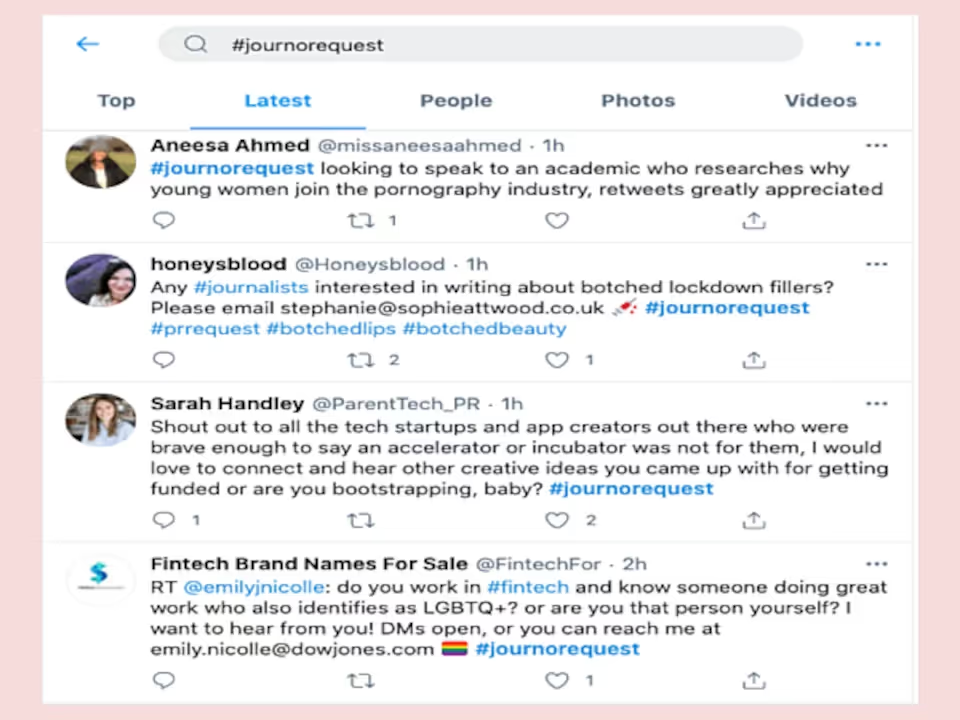
A Practical SEO Guide to Digital PR
Learn how you can use the latest digital PR techniques to increase the exposure of your brand by gaining online coverage on well known sites, social media platforms and generating high quality links and referral traffic. This practical step-by-step guide gives you the essential information you need to start this process yourself or at the very least brief a digital PR agency.

A Practical SEO Guide to International SEO
In this in-depth gudie to international SEO, our expert SEOs take you through the key differences and scenarios you will encounter when optimising a single mulitlingual site or multiple sites in different countries. It covers practical tips and advice and lessons from the trenches about Local SEO vs International SEO, Market Entry strategies and common pitfalls and how to mitigate (if not avoid) them.
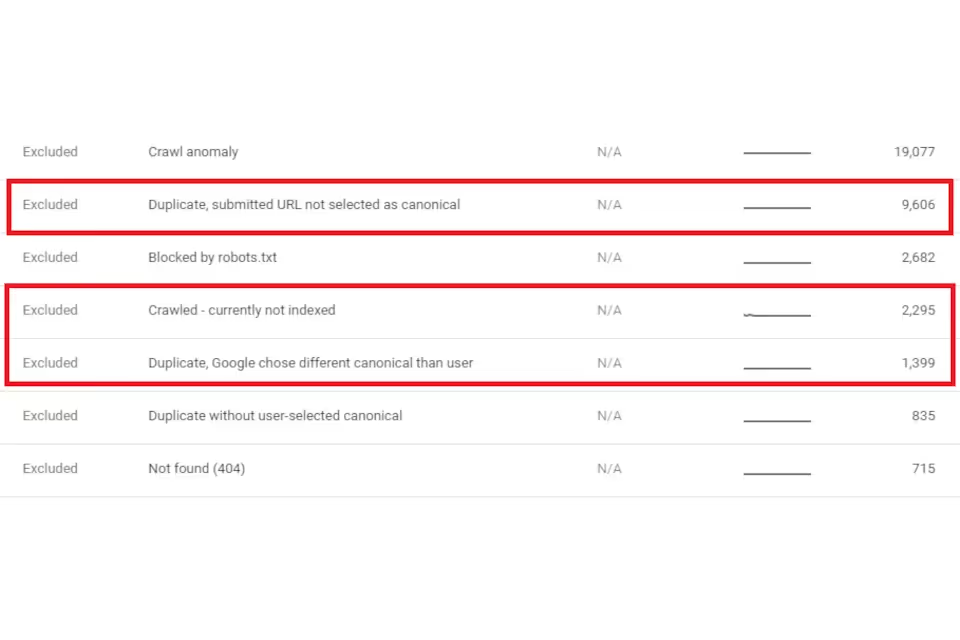
A Practical SEO Guide to Technical SEO
Technical SEO is the foundation on which successful SEO campaigns are built. If you're not a web designer or developer then this guide to technical SEO is for you. You can learn the key technical issues that expert SEOs look to resolve to prevent issues for users and search engines.
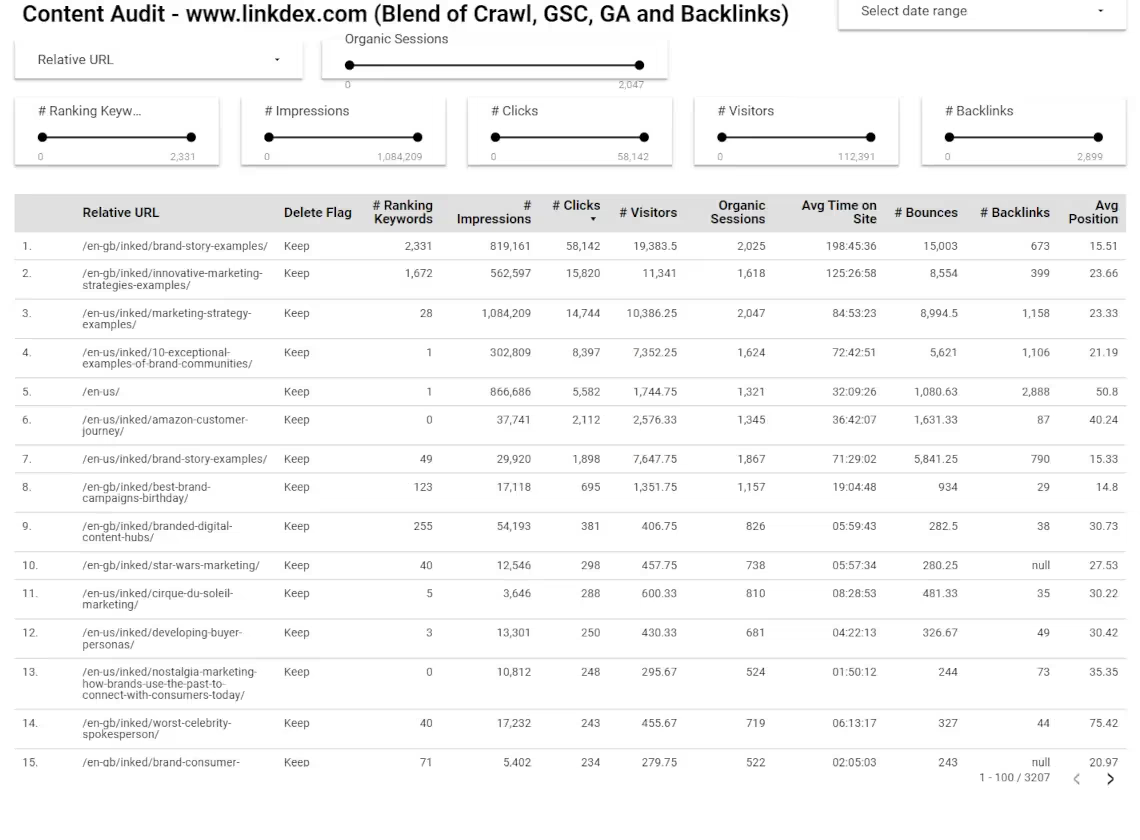
A Practical SEO Guide to Content Auditing
Want to know how to conduct an SEO content audit on your website? Our guide, carefully put together by three great SEOs covers everything you need to know to get started. From properly understanding the performance and the potential of your pages, to evaluating user intent to see how well your existing content matches the type of content Google prefers. Authoritas can now even automate the data collection and presentation of the data needed to perform a content audit.
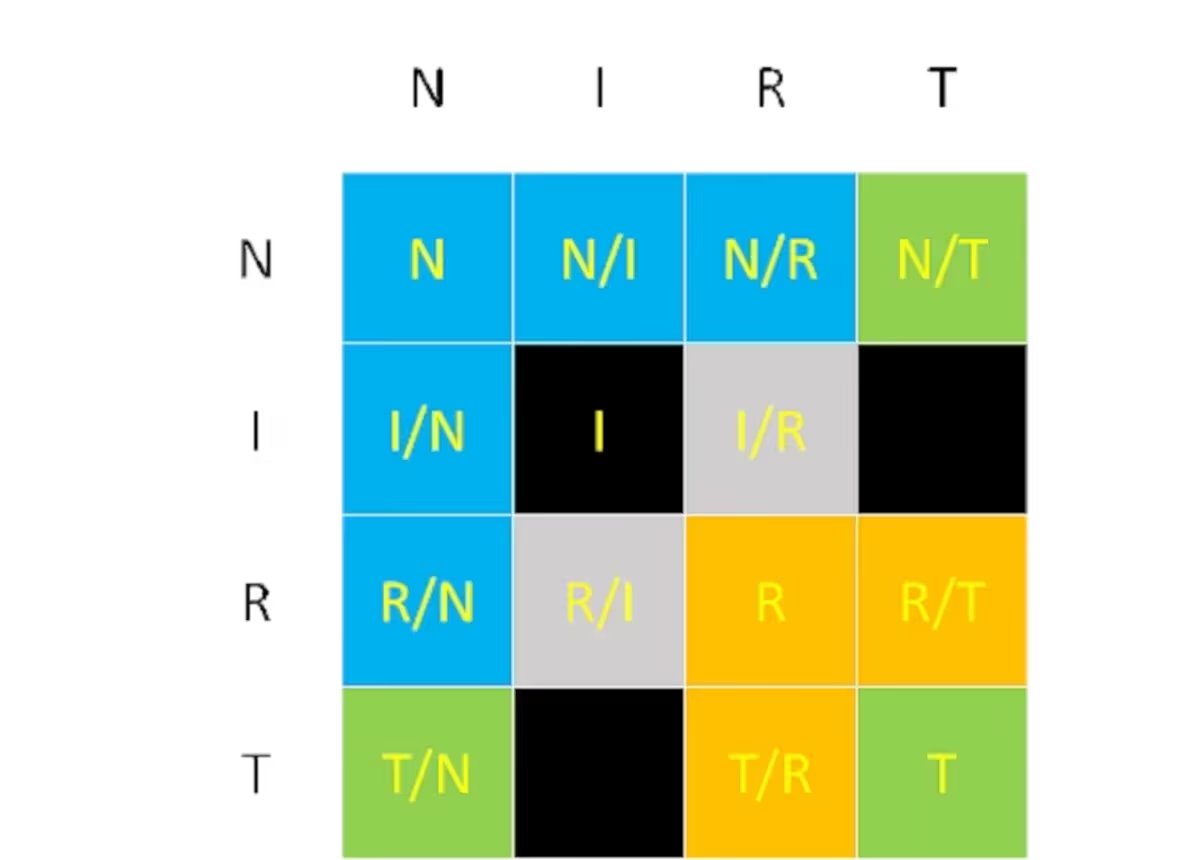
A Practical SEO Guide to User Intent
The whole SEO industry has been debating how best to understand what the searcher's intent is for the past few years. In this guide, we look at answering practical questions around user intent, what it is, how to analyse it and what to do with your findings.

A Practical SEO to On-Page SEO
This guide goes into detail about how to improve your On-Page SEO strategy with a set of actionable topics and tasks for you to consider when optimising your pages for users and search engines.

A Practical SEO Guide to Competitor Auditing
This guide helps you take a systematic look at all the key elements of competitive analysis for SEO. It covers, how to define your market, how to analyse your competitors' performance, competitive gap analysis, SWOT analysis, SERP analysis and how to set goals for your SEO project.

A Practical SEO to Content Strategy
Setting a sound plan for your content marketing efforts is the key to success. In this guide to SEO content strategy, three experienced SEOs share their advice on content strategy for Local SEO, how to create content strategies for eCommerce sites and what your content strategy should look like for your blog.